Modern research into functional materials has greatly benefited from the availability of large scale scientific experimental facilities such as synchrotrons and x-ray free electron lasers. However, one of the major obstacles to fully utilizing these facilities and the associated state-of-the-art experimental techniques is that data is acquired at a rate that is, by many orders of magnitude, faster than can be analyzed using standard methods. This issue exists in many types of modern research areas including high throughput combinatorial methods for new materials discovery, rapid measurements with instruments of high repetition rate, and surveys of large-scale samples using high resolution probes. How can we efficiently extract the scientifically important information from the big data while minimizing the requirement of human interaction poses a frontier challenge.
One example to show the importance of supervised and unsupervised scientific big data mining is in the spectro-microscopic investigation of new materials. Our earlier developments in spectro-microscopy have made it possible to acquire nanoscale, spatially resolved spectroscopic data at a rate of over 6,000 spectra per second, which makes it practically impossible for the researcher to interact with every spectrum as it is recorded. On the other hand, it is risky to simply select a subset of the big scale scientific data for detailed analysis. This is because the unknown/new material phases, which are functionally important in such hierarchically complex and structurally heterogeneous systems, are often minority components that are spatially segregated into a few small localized regions such as at the grain boundaries and/or the reaction front. Automatic classification of the large scale scientific data would greatly improve the overall research efficiency by guiding the researchers to the hidden features that are potentially of scientific importance as identified by means of supervised and unsupervised big data mining.
Our group has been devoting our effort into this field. We apply advanced computing methods in the analysis of synchrotron data for effective and efficient information mining. More specifically, we use machine learning tools to identify scientifically important subsets of the data with several successful case studies in the field of battery research.
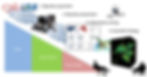